Customer churn is one of the key challenges facing organizations in today’s highly competitive environment. In order to effectively combat customer churn, companies need to find answers to two key questions: which customers are at risk of churning, and what actions can be taken to stem the process.
With help comes a combination of two methods from the arsenal of data science: prediction and clustering (segmentation), which together increase the effectiveness of retention efforts.
I have already addressed the issue of prediction of the threat of customer churn in an article in the We Love Data So Let’s Date series. Due to the importance of the topic and the interest it has generated, I decided to deepen the issue and present an approach in which, by combining two machine learning methods, we can significantly facilitate the implementation of data-driven anti-churn activities.
Prediction and segmentation as a weapon in the fight against customer churn
The basic tool in countering customer churn is the predictive model. It allows predicting the probability of a particular consumer leaving. However, this may not be enough. Even the best model and the most accurate prediction will be of no use if we do not take appropriate action based on the information received.
Proper interpretation of the data allows us to plan activities and take actions – such as sending an sms message to customers or offering a discount on selected products. We then measure the impact of the action (effect) on consumer behavior and their propensity to abandon further use of the offer. Measuring and observing consumer behavior provides a source of new data that the predictive model can use. Thus the cycle closes, and this is illustrated in the diagram below
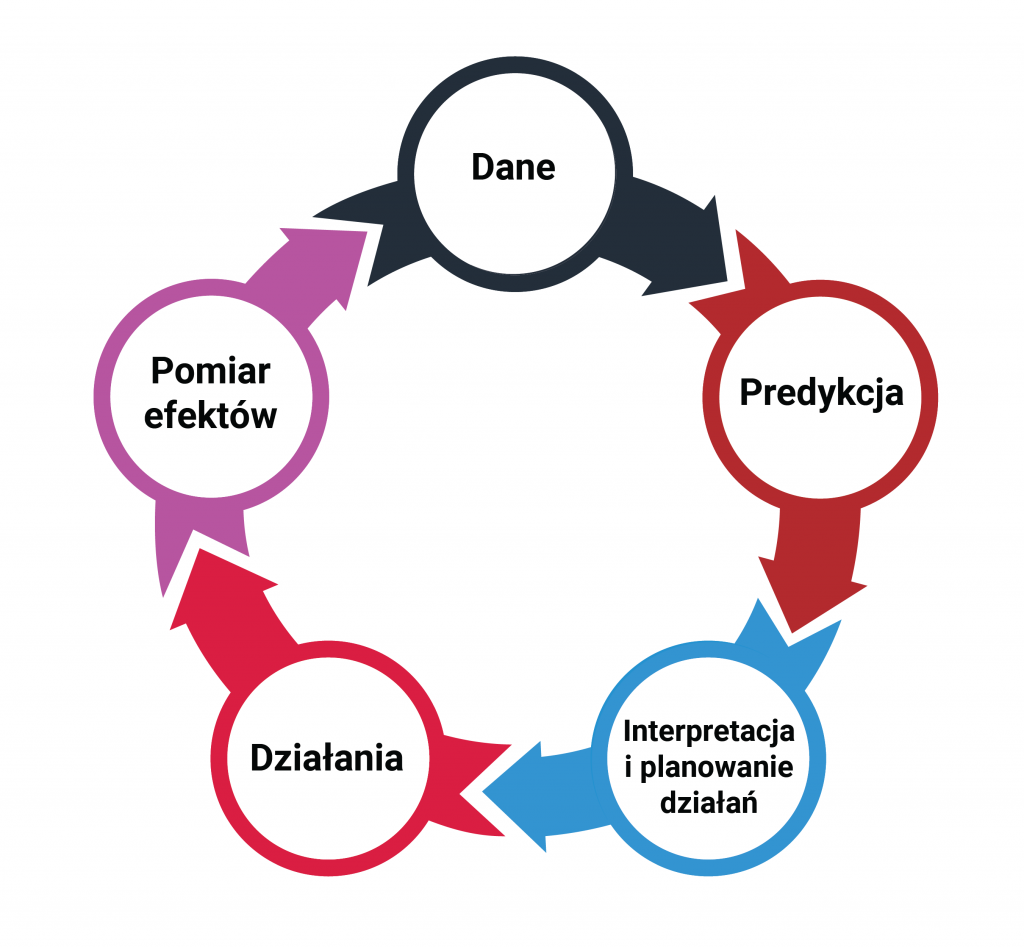
The predictive model indicates the likelihood of a specific customer churning. This allows us to prioritize tasks and use the usually limited resources on consumers most at risk of leaving. Information that a consumer has a 75% probability of leaving within the next quarter helps us decide that “something” must be done about it quickly. However, does it provide the knowledge of what to do? How does a company know what action to take? The key is to interpret the data correctly.
The ideal situation would be to be able to take action personalized to the individual consumer. That is, each customer would receive a unique customized offer tailored to their needs and problems. Predictive models built on the basis of appropriately selected machine learning methods make it possible to create an individual profile of each consumer. In addition, they indicate specific factors that in his case are associated with a higher risk of leaving. Despite advances in the area of hyperpersonalization of marketing activities, it is still not yet achievable on a large scale for many organizations. Not all activities can be automated as easily. For example, creative, content production or offer construction can be a limitation.
The solution in such a case is an approach based on advanced segmentation. However, it is definitely not about classic segmentation, which uses only basic variables like age or gender. They do not sufficiently differentiate the base, and the real dividing lines run quite elsewhere. It is important, therefore, that it be a behavioral segmentation, in which we look for similarities in customer behavior, taking into account the same broad aggregate of descriptive variables that was used to build the predictive model. In order to perform such a comprehensive segmentation, clairvoyant machine learning algorithms are required.
The procedure may look as follows:
- The predictive model allows us to identify the group of customers most at risk.
- We decide that we have the resources to act against the 20% of the most at-risk customers.
- We select these customers and, using a clustering model, divide them into segments. Their number should be a product of the model’s indications and the resources we have to serve them (usually it will be several – a dozen).
- We interpret the segments and plan actions.
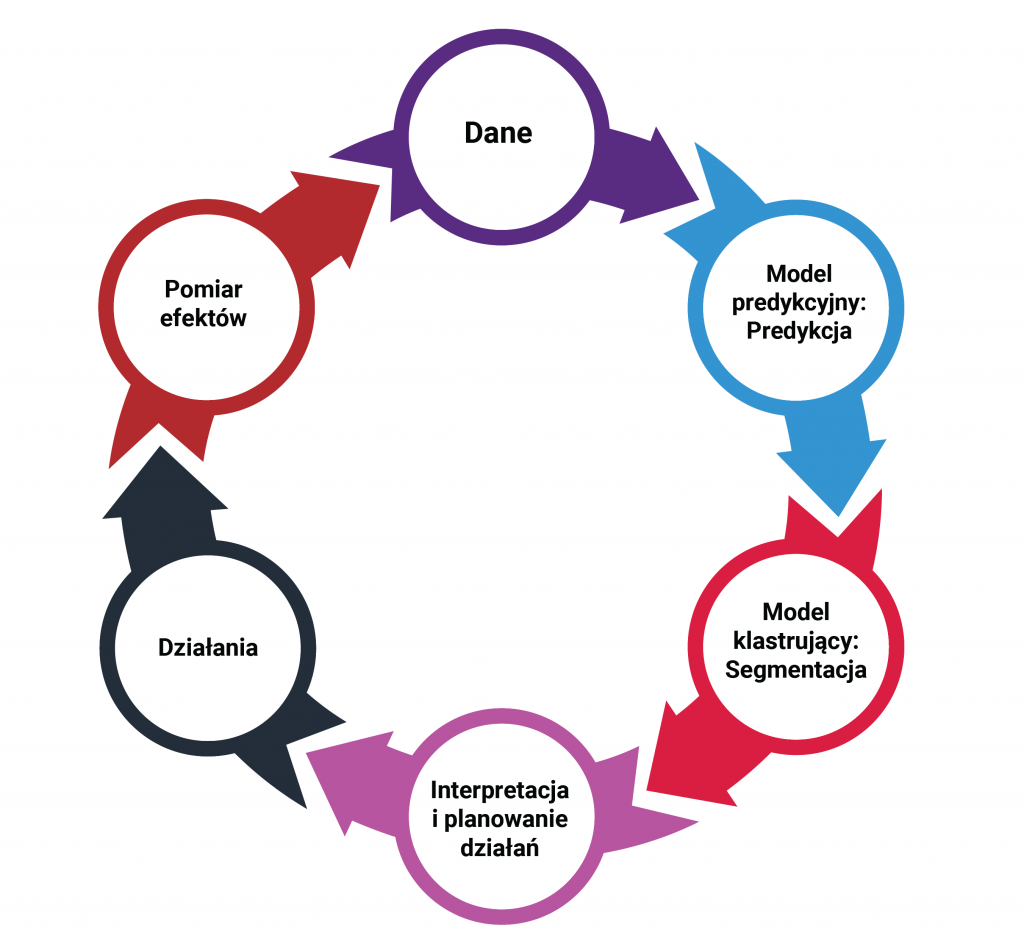
The proposed method makes it possible to put into practice predictive modeling and the in-depth understanding provided by data science analysis of consumers at risk of leaving. The approach will also find application in companies not yet technologically and organizationally ready for fully automated hyperpersonalization efforts.
.