Finding a compromise between maximizing profits and reducing costs is not an easy task for marketers planning marketing campaigns. For campaign ROI it is crucial to choose the right group to target. With help comes uplift modeling, which examines the likelihood of customer purchase.
The middle of summer. A bit of a “dead” season. Conversations in the marketing department of one of the largest retailers in Poland concern not only impressions from vacations, but also how to stimulate sales a little. One of the employees suggests running a text message campaign. There is a consumer base that can be communicated with. There is even quite an attractive offer that can be written about. Nothing else to do but send it. A problem arises, though. The end of the financial year is approaching, so there is not much money left in the budget. Enough to hold a mailing to at most one-fifth of the base. Enthusiasm has subsided – there will be no fireworks. But what can be done to make the most of the limited budget and maximize the chances of achieving a noticeable effect? Someone comes up with an idea to get on to friendly data science consultants. Time is short and one should to act fast, but the experienced Data Science Logic team takes up the challenge.
Can we predict the purchase?
We can describe consumers in the database on nearly 200 variables: in terms of transaction history, assortment purchased, price sensitivity, propensity to buy online, interaction with marketing communications, and visits to the retailer’s website. Analysts build a scoring model predicting the likelihood of interest in the promoted assortment for each consumer who could potentially be contacted.
The available budget will be divided into two parts. Half of the consumers will be selected in the existing way. The other part will be the 10% of the most interested consumers according to the model’s prediction. Additionally, from among all those qualified for the mailing, a control group will be drawn, which will not receive the message. This division allows us to measure the effectiveness of the two targeting methods and the effect of the communication itself.
Results: conversion rate in the group selected by the model is nearly 3 times higher than in the group selected by the previous method. The results speak for themselves. Data science wins. Or does it?
Are we sure we are looking at the right indicator?
The conversion comparison shows that the model correctly predicted a group of consumers above average interested in buying. But were not these customers who would have completed the transaction anyway even without the text message? What was the actual impact of the mailing on their tendency to buy? We can find answers to these questions by making a comparison with a control group randomly excluded from the communication. It shows that the difference between the conversion in the entire messaged group and the conversion in the control group was about 1.8 percentage points. The difference is still in favor of the model, but is no longer so spectacular. This means that some of the consumers identified by the model were already sufficiently interested in the purchase before the communication, and there was no need to stimulate them additionally. So how can we classify consumers in terms of their expected response to a marketing communication?
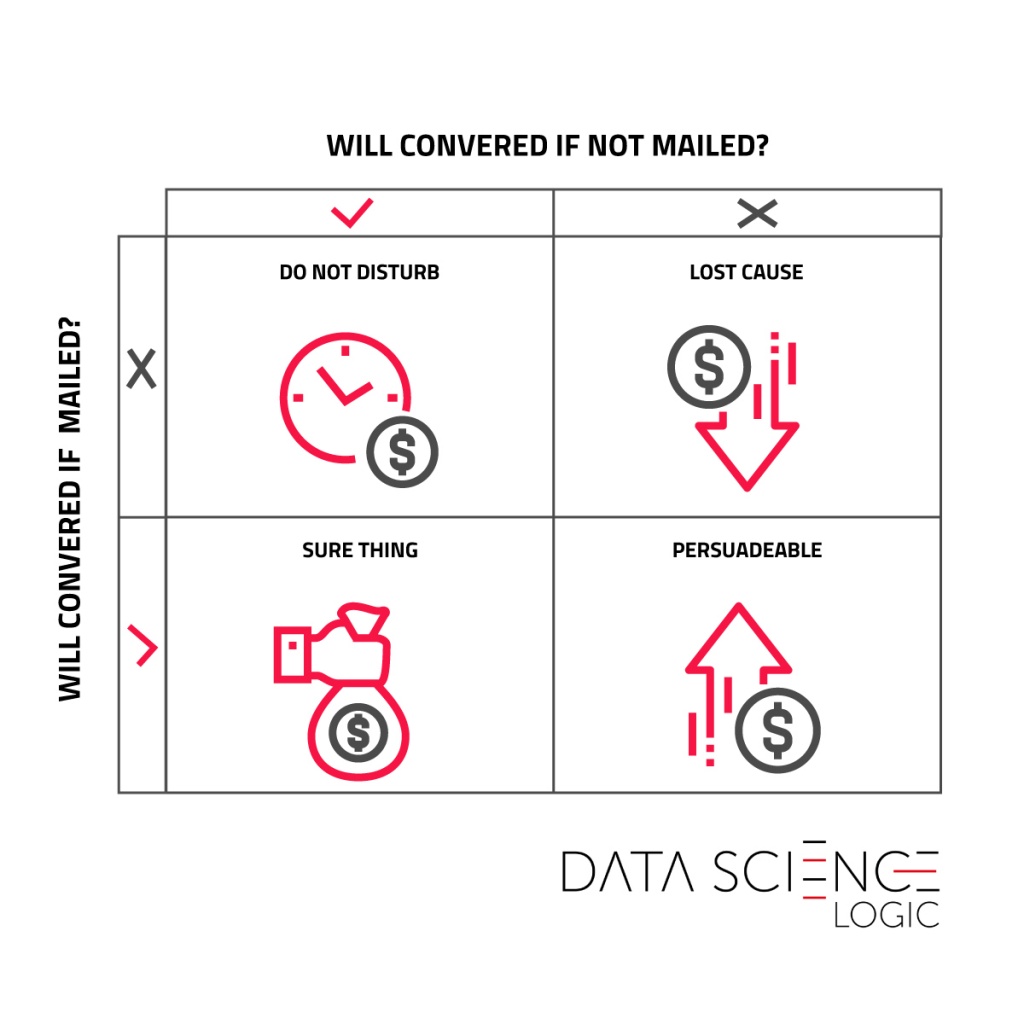
The top left part is the people ‘Do not disturb‘ group, who would have been interested in the transaction, but disturbed by the unwanted communication abandon the purchase. The ‘Lost cause‘ section are consumers who we cannot convince to buy, even with a planned campaign. The ‘Sure thing‘ group are people willing to buy even without communication. Finally, the lower right square ‘Persuadable‘ group that is not yet convinced to buy and the campaign stimulus is able to impact the decision. So we have one group that is worth communicating and three that are not. But how to predict who is in this profitable group?
The uplift model
Again, data science comes with help. It is possible to build a model that will predict not so much the propensity to buy as the change of this propensity under the impact of communication. This is the so-called uplift model. The prediction of the model allows to rank consumers from those with the highest increase in purchase probability to those with the lowest (or even negative) change in interest in the transaction. Data scientists build an uplift model based on the data collected in the first mailing. Its application in the next experiment brings further increase of uplift – by almost 0.4 percentage points in comparison to the group selected by the response model. Seemingly little, but with the appropriate scale of the database translates into a significant number of additional transactions generated. Compared to the previously used selection methods, the response model generated 10% more additional sales, and the most advanced uplift model generated almost 30% more.
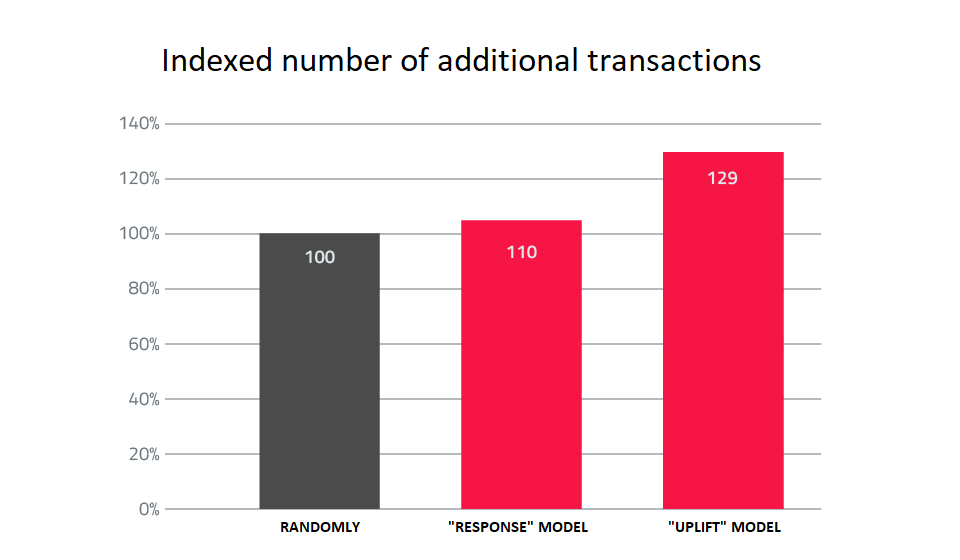
What we buy, spending the budget on communication with consumers, are in fact additional conversions that would not be achieved if it were not for the campaign. Properly selecting the group communicated, we can with the same budget generate significantly more incremental purchases. Uplift predictive modeling available among data scientists tools can be a significant help here.